Joker123: Daftar Joker Gaming dan Link Situs Joker388 Online
Joker123: Daftar Joker Gaming dan Link Situs Joker388 Online
Joker123 adalah platform online yang menawarkan beragam permainan judi slot populer, mesin slot inovatif serta permainan realitas virtual modern. Didukung oleh teknologi mutakhir yang dilengkapi dengan grafis serta efek suara memukau, joker123 melayani pemain dari semua level, memastikan bahwa setiap orang dapat menemukan permainan pilihan mereka dan menikmati hiburan mendebarkan tanpa henti. Salah satu fitur utama joker123 adalah antarmukanya yang ramah pengguna. Menavigasi platform ini sangat mudah, memungkinkan pemain dengan mudah menjelajahi berbagai pilihan permainan dan memilih permainan diinginkan hanya dengan beberapa klik. Selain itu, joker123 memastikan pengalaman bermain game dengan lancar di berbagai perangkat, mulai dari komputer desktop hingga ponsel cerdas maupun tablet, memungkinkan pemain untuk menikmati permainan favorit mereka kapan saja, di mana saja. Selain pilihan permainan mengesankan dan antarmuka ramah pengguna, joker123 menonjol dari para pesaingnya dengan menawarkan bonus promosi menarik untuk meningkatkan pengalaman bermain. Setelah mendaftar, pemain akan disambut dengan bonus member baru, yang semakin mendorong eksplorasi pemain terhadap katalog game yang luas.
Selain itu, Joker123 secara rutin memperbarui promosinya, memberikan peluang untuk menang besar dan menikmati sensasi kemenangan. Bonus ini tidak hanya meningkatkan tingkat kegembiraan tetapi juga memberi peluang tambahan untuk meraih kesuksesan besar. Keamanan adalah hal paling penting dalam dunia perjudian online, joker123 memahami hal ini. Dengan teknologi enkripsi canggih serta langkah-langkah keamanan kuat, joker123 memastikan bahwa informasi pribadi keuangan pemain tetap terlindungi setiap saat. Komitmen terhadap privasi dan keamanan ini telah membuat joker123 mendapatkan reputasi sebagai situs judi slot online tepercaya, memberikan ketenangan pikiran bagi para pemain.Selain itu, platform ini menyediakan berbagai alat dan sumber daya untuk membantu pemain memantau serta mengendalikan kebiasaan perjudian mereka, memastikan pengalaman bermain nyaman dan aman bagi semua orang. Joker123 menawarkan petualangan bermain game slot tak tertandingi. Seiring dengan berkembangnya komunitas slot online, joker123 tetap menjadi yang terdepan, terus berkembang dan berinovasi untuk menghadirkan pengalaman bermain terbaik.
Game Slot Joker123 Gaming Dengan Bonus Jackpot Progresif Tertinggi
Slot joker123 gaming dikenal sebagai salah satu platform perjudian online terbaik yang menawarkan berbagai permainan slot joker menarik dan mengasyikkan. Salah satu kelebihan utama dari joker123 gaming adalah menawarkan bonus jackpot progresif tertinggi. Jackpot progresif adalah jenis bonus yang terus meningkat seiring dengan setiap taruhan yang ditempatkan pada mesin slot tertentu. Hal ini berarti bahwa semakin banyak orang memainkan permainan joker123 gaming tersebut, semakin tinggi juga jumlah hadiah yang akan diberikan kepada pemenang jackpot. Slot joker123 gaming dengan bonus jackpot progresif tertinggi tidak hanya memberikan kesempatan bagi pemain untuk memenangkan hadiah besar, tetapi juga menawarkan tingkat kegembiraan tak terbandingkan. Pemain akan terus merasa terpikat dengan kesempatan untuk menang besar, dan sensasi itu tidak akan pernah berhenti. Berikut daftar game slot joker123 gaming dengan bonus jackpot progresif tertinggi:
- Roma Slot - Jackpot 98.45%
- Sizzling Hot - Jackpot 97.85%
- Roma Legacy - Jackpot 97.77%
- Golden Dragon - Jackpot 97.48%
- Mayan Gems - Jackpot 96.53%
- Thunder God - Jackpot 96.29%
- Wealth God - Jackpot 96.15%
- Lucky God Progressive - Jackpot 95.29%
Keuntungan Bermain Slot Joker Gaming di Situs Joker388 Terpercaya
Perjudian online semakin populer di era digital ini. Salah satu bentuk perjudian online yang banyak diminati adalah permainan slot. dalam dunia perjudian online, terdapat banyak situs penyedia permainan slot joker gaming. Salah satu platform terpercaya yang bisa Anda pilih adalah situs joker388. Bermain slot joker gaming di situs joker388 memiliki banyak keuntungan. Pertama-tama, situs ini menyediakan berbagai macam permainan slot menarik. Anda dapat menemukan berbagai tema dan fitur menarik dalam permainan ini. Apakah Anda menyukai tema kasino klasik atau preferensi modern, slot joker388 memastikan permainan sesuai dengan selera Anda. joker388 slot juga memiliki layanan pelanggan yang responsif dan ramah. Mereka siap membantu para pemain dalam menyelesaikan masalah atau menjawab pertanyaan dengan cepat. Jika Anda mengalami kendala saat bermain slot joker gaming online, Anda dapat menghubungi tim dukungan pelanggan mereka dan mendapatkan bantuan segera.
Dengan semua keuntungan yang ditawarkan oleh situs joker388, tidak heran jika semakin banyak orang tertarik untuk bermain slot joker gaming 123. Selain keuntungan-keuntungan tersebut, slot joker388 juga telah terbukti terpercaya dan memiliki reputasi baik di dunia perjudian online. Sebelum bermain slot joker gaming di situs joker388, pastikan Anda telah mendaftar dan memiliki akun. Setelah itu, Anda dapat mulai menikmati berbagai permainan slot yang menyenangkan dan menghasilkan keuntungan. Jika Anda mencari pengalaman perjudian online yang aman dan mengasyikkan, bermain di situs joker388 adalah pilihan tepat. Keuntungan bermain slot di situs Joker388 terpercaya sangatlah banyak. Dari berbagai macam permainan menarik hingga bonus dan promosi menguntungkan, tidak diragukan lagi bahwa situs ini adalah salah satu yang terbaik di industri perjudian online. Jadi, jangan lewatkan kesempatan untuk merasakan sensasi perjudian online yang seru dan menghibur di situs joker388.
Info Situs Joker123
|
π Roma Slot, π Sizzling Hot, π Roma Legacy, β‘Golden Dragon |
Nama Situs
|
π° JOKER123 SC77 |
Winrate Slot
|
97.88% |
Deposit Via Bank
|
π BCA, π₯ BRI, π₯ BNI, π₯ MANDIRI π₯CIMB. |
Minimal Deposit
|
π° Min Deposit Rp 10.000 |
URL Situs
|
Kunjungi Situs |
Share
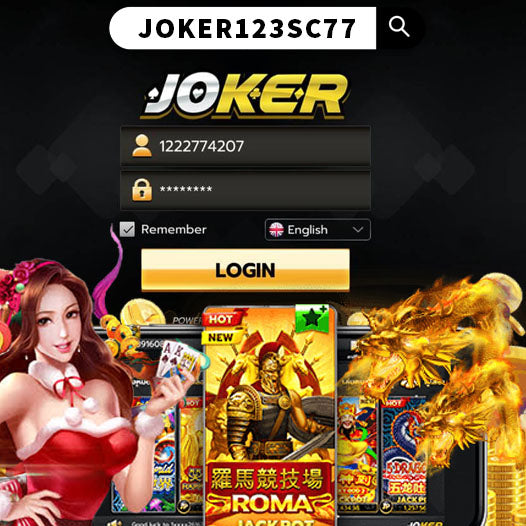